The non-profit LAION publishes the current best open-source CLIP model. It could enable better versions of Stable Diffusion in the future.
In January 2021, OpenAI published research on a multimodal AI system that learns self-supervised visual concepts from natural language. The company trained CLIP (Contrastive Language-Image Pre-training) with 400 million images and associated captions.
CLIP trains an image encoder and a text encoder in parallel to predict the correct image and caption pairings from a set of training examples.
OpenAI released the larger versions of CLIP in stages leading up to April 2022, and parallel efforts to reproduce CLIP emerged in the open-source community.
CLIP takes a central role in generative AI models.
Once trained, CLIP can compute representations of images and text, called embeddings, and then record how similar they are. The model can thus be used for a range of tasks such as image classification or retrieving similar images or text. OpenAI used CLIP to filter images generated by DALL-E 1 by quality, among other things.
In the generative AI models for images created after DALL-E 1, CLIP often takes a central role, for example in CLIP+VQGAN, CLIP-guided diffusion, or StyleGAN-NADA. In these examples, CLIP computes the difference between an input text and an image generated by, say, a GAN. The difference is minimized by the model to produce a better image.
In contrast, in newer models, such as DALL-E 2 or Stable Diffusion, CLIP encoders are directly integrated into the AI model and their embeddings are processed by the diffusion models used. Researchers from Canada also recently showed how CLIP can help generate 3D models.
LAION releases powerful OpenCLIP
Now, the non-profit LAION is releasing three major OpenCLIP models. LAION (Large-scale Artificial Intelligence Open Network) trained two of the models with funding from Stability AI, the startup behind Stable Diffusion.
One of the models was also trained on the JUWELS Booster supercomputer. The research network previously published primarily open-source datasets such as LAION-5B, a gigantic image dataset with associated captions (5.8 billion image-text pairs).
The OpenCLIP models now released (L/14, H/14, and g/14) are among the largest and most powerful CLIP open-source models to date. For training, the team relied on the slightly smaller LAION-2B dataset.
The H/14 model achieved a top-1 accuracy of 78.0 percent in the ImageNet benchmark and an accuracy of 73.4 percent in the zero-shot image retrieval benchmark MS COCO at Recall@5, making it the best open-source CLIP model as of today.
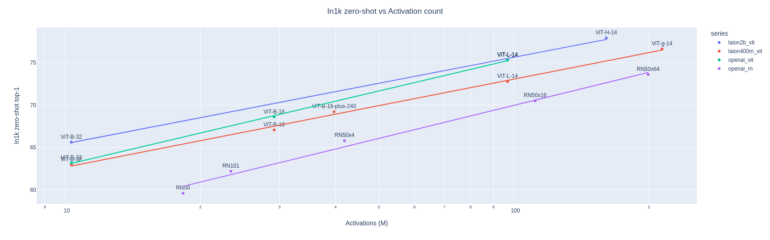
LAION's training also shows that CLIP models, like other Transformer models, scale in capability with training data and size.
LAION's models could enable better generative AI models
The models were trained on up to 824 Nvidia A100 GPUs with 40 gigabytes of VRAM. During training, the team uncovered and solved several interesting problems, including identifying faulty GPUs, the beneficial role of large batch sizes in training, and the impact of different floating-point formats on training stability.
LAION's new CLIP models can now be used for numerous applications at no cost. Stable Diffusion can also benefit from the new models, the team said. Proving CLIP's scaling properties also opens the way to further improvements, they said. For example, LAION plans to integrate and scale a multilingual text encoder in CLIP.
Away from image processing, the idea behind CLIP could be extended to other modalities, such as text-to-audio alignment, the team writes. The project is already underway and is named CLAP. The OpenCLIP models are available via GitHub and at HuggingFace.